Policy consultation response: Verra's Long-Term Reversal Monitoring System
.avif)
Verra is developing a system to monitor forest projects beyond the end of their crediting period and address any reversals. Sylvera supports this proposal and suggests some current best practice methodologies for ongoing remote forest monitoring and carbon quantification.
Sylvera endorses the concept of a Long Term Reversal Monitoring System (LTRMS) to address concerns around reversal risk in Verified Carbon Standard (VCS) projects during the post-crediting period. Nature-based carbon credits have inherent permanence risks, and mechanisms such as the LTRMS must be put in place in order for voluntary carbon markets (VCMs) to function with high integrity. Permanence and reversal risk are key components that must be continuously monitored and quantified in a systematic, cost-efficient, and democratized way to ensure the validity of net zero claims when offsets are used to compensate for emissions. After a loss event is detected and quantified, the entity administering the LTRMS would then account for the reversal and cancel buffer credits accordingly.
Should Verra monitor VCS Agriculture, Forestry and Other Land Use (AFOLU) projects for reversals during the post-crediting period? If so, why, if not, why not?
Yes, Verra should monitor VCS AFOLU projects for reversals during the post-crediting period. Permanence is one of the most significant criticisms of nature-based carbon crediting projects, especially relative to technological solutions. Long-term monitoring and quantification of loss events, and the corresponding buffer credit cancellation and accounting, is required to support high-integrity VCMs. Additionally, the LTRMS would allow Verra to better calibrate their risk buffer percent thresholds to ensure the buffer pool is sufficient to compensate for long-term reversals. This would also bolster the proper functioning and integrity of AFOLU projects in VCMs.
What would be the key opportunities, benefits, challenges, and risks of Verra doing this?
The LTRMS would improve confidence in the integrity and credibility of AFOLU projects, which would then stimulate further investments in (i) carbon credits, (ii) natural capital, and (iii) the flow of finance toward green development. Moreover, the LTRMS should be implemented in a cost-efficient manner by leveraging remote sensing and machine learning technologies, and the LTRMS results should be made publicly available to bolster confidence and credibility in the market.
Sylvera’s major concerns are around the extreme, non-linear feedback loops associated with climate change and the potential shortfall of buffer pool credits available to account for long-term reversals. The LTRMS is an excellent first step, but we do see additional opportunities to further enhance and build trust in AFOLU projects through climate risk modeling and having the results of those simulations guide the risk buffer withholding percentages for ongoing projects to mitigate and minimize reversal risks.
What types of VCS AFOLU projects could currently be monitored for reversals effectively and efficiently using available remote monitoring approaches? How are remote monitoring technologies expected to evolve in the near future, and should this enable monitoring for additional AFOLU project types and activities (e.g., degradation)? If so, which and by when?
Sylvera is currently able to monitor Above-Ground Biomass (AGB) reversals in Reducing emissions from deforestation and forest degradation (REDD+) projects. We are also actively developing models to detect reversals in Improved Forest Management (IFM) and Afforestation, Reforestation and Revegetation (ARR) projects.
We expect that remote sensing technologies will evolve in the coming years to enhance existing detection models, for example to more accurately assess forest degradation and other small-scale activities. Degradation is currently challenging to monitor precisely but is proving a very significant source of emissions. A recent study found that the Amazon rainforest is losing more carbon from degradation than deforestation. We are looking forward to incorporating new satellite data opportunities such as BIOMASS, available by the end of this year, and NISAR, in 2023, in our carbon credit ratings. Further, we also expect public and commercial data to increase in resolution, to become more accessible, and have better spatial and temporal coverage which will improve the accuracy of monitoring.
Soil carbon:
To date, the most significant challenge of remote sensing is its application to Below-Ground Biomass (BGB) pools and soil organic carbon. These store up to 80% of terrestrial carbon, and are vulnerable to climate change, degradation and other disturbance. Current soil models have extremely high uncertainties and most soil is not directly observable with remote sensing. However, there are several promising avenues of research, many of which can be used in conjunction.
Direct measurements:
The most promising direct satellite measurement for soil carbon is visible, visible near-infrared (vis-NIR) spectroscopy and midinfrared (MIR) (vis-NIR/MIR) spectroscopy. Such sensors have been used in recent research when doing direct measurements of soil samples, with equally good results as traditional methods when deciding the soil carbon content. When taking the step up to satellite sensors the spectra is not clear enough yet, but there is hope that it will improve with new, hyperspectral, high-resolution sensors.
Peatlands offer further possibilities for direct measurements. For example, there have been efforts to use Synthetic Aperture Radar (SAR) to measure the "curvature" of a peat bog with high resolution in the z-direction. By tracking the growth or shrinkage of the peat, it is possible to infer water content, which indicates the decomposition rate. There may also be a correlation between the curvature and the depth of the peat.
There is also interest in the use of ground-penetrating radar to measure the depth of peatland, as theoretically a clear change in density should exist at the border between peat and the ground below.
Hyperspectral sensors can be used to determine peatland extent, as most are largely covered by a sphagnum moss, which has a specific spectral signature.
Indirect measurements
An alternative to directly measuring soil carbon is to use "proxies" relating to soil carbon content. For example, a soil with high carbon content usually contains more moisture. Another example could be to relate vegetation growth indices, such as normalized difference vegetation index (NDVI), with soil carbon or below ground biomass (BGB).
Digital Soil Mapping (DSM) combines multiple proxies and direct measurements and uses ML methods to correlate these covariates with ground truth measurements of soil carbon. This generates a predictive model which can be applied to any area. A good, global example of this is the SoilGrids project.
Similar DSM methods have been developed on a smaller scale for peatlands in Southeast Asia. This is an emerging research field, but with accurate ground truth training data, Sylvera could envisage training DSM models for specific biotopes and regions.
Agricultural soil carbon presents further opportunities for remote sensing of soil carbon. It is possible to identify certain crop species with hyperspectral sensors. In-situ measurements of the BGB of specific crops would allow inferences of BGB across croplands. Satellite data can also be used to monitor drivers of change in soil carbon, such as agricultural practices. This could even include hyperspectral analyses with a high time resolution to monitor spikes in local methane emissions when organic fertilizer is applied.
Would the LTRMS and associated proposed periodic adjustments to the VCS withholding percentages (based on monitored losses by risk category) increase confidence in the long-term resilience of the AFOLU buffer pool and issued project credits?
The LTRMS would absolutely increase confidence in the long-term resilience of the AFOLU buffer pool and issued credits, provided that the LTRMS results had a direct feedback loop to the withholding percentages and risk buffer allocations for ongoing projects and was also informed by the most up-to-date scientific evidence. For example, if the LTRMS detects extreme losses for the majority of projects that averaged ~40% per project, and the risk buffer allocations for ongoing projects remain at 10% to 20% then the prescribed withholding percentages should be increased to be in line with the results from the LTRMS. This feedback loop between the LTRMS and Verra’s established withholding percentages would increase confidence in AFOLU offset projects and the security that the buffer pool provides as an insurance mechanism. Furthermore, Sylvera recommends that the LTRMS results are transparent and publicly available to further bolster confidence and trust in the market.
Additional climate risk modeling that highlighted non-linear feedback loops, in conjunction with the LTRMS and scientific evidence, would also enhance confidence in AFOLU credits.
Over how long a period should Verra monitor reversals after the project crediting period ends (e.g., 50 years, 100 years)?
In terms of increasing confidence in the permanence of AFOLU projects, longer periods should be encouraged. 100 years aligns with IPCC timescales.
How frequently should Verra aim to monitor for loss events (e.g., quarterly, bi-annually)?
Quarterly monitoring is viable but would instead recommend annual or bi-annual monitoring initially and then plan to phase in more frequent updates, for example, quarterly, monthly, and so on, over time. Seasonality must be taken into account in seasonal forests to ensure it is not considered forest loss, for example by comparing to the same period in previous years. Increased frequency of monitoring would be welcome to understand these patterns. For a higher monitoring frequency, SAR data is more appropriate and the number of satellites launching in the future is expected to increase.
If Verra ceases to operate or manage the LTRMS prior to the end of this monitoring commitment how could environmental integrity be maintained (e.g., through cancelation of all buffer credits associated with the project)?
Verra could assign operatorship of the LTRMS to an earth observation company that is equipped to perform this continuous monitoring in a cost-efficient, transparent manner. Should the LTRMS cease to be in operation, by Verra or its assignee, then the buffer credits should be canceled or held in reserve for other AFOLU projects that have not yet reached the end of their monitoring period.
What best practices, standards and/or guidance should the LTRMS follow? Are there potential limitations to the applicability, accuracy, reliability and credibility of a remote monitoring approach for identifying AFOLU reversals?
The LTRMS should be performed via remote sensing technologies which is far less costly than the current verification procedures. The reduced costs of the LTRMS would eradicate barriers to participants such as community-led or indigenous groups. The LTRMS should be transparent and results should be made publicly available to bolster confidence in the market.
The current data can be easily used for large deforestation or activities such as fires alert systems. The best practice is not only to use the best available data available for each year but also to combine different remote sensing modalities to overcome any uncertainties and increase the robustness of models and monitoring systems.
There exist a number of limitations regarding the monitoring of forestry projects using remote sensing. Detecting small-scale activities such as degradation, selective harvesting, or any activity at a smaller scale is limited by the resolution of the Earth Observation data, see question 3. If only using optical data, cloudy areas can limit monitoring. This can be mitigated by using SAR or lidar data.
When using machine learning to interpret satellite images, accurate training data is essential. To quantify reversal, changes in forest cover detected through remote sensing must be interpreted to infer changes in above ground biomass (AGB) carbon stock, and therefore carbon emissions. The allometric models in widespread use now have high uncertainties and have been shown to be biased to underestimate AGB by up to 40%.
Sylvera has developed a state-of-the-art capability for accurate and timely generation of in-situ training data using multi-scale lidar (MSL) measurements, to construct AGB maps for large areas, tens to hundreds of thousands of hectares, of forest.
These MSL measurements are collected from both the ground and air: terrestrial laser scanning (TLS) data are collected from sample plots, while unoccupied aerial vehicle laser scanning data are collected in slow- and fast-flying configurations, unoccupied aerial vehicle laser scanning (UAV-LS) and airborne laser scanning (ALS), respectively. This assemblage of data, collected across spatial scale, from the individual tree, to region, combined with novel processing algorithms, provides the unprecedented ability to accurately estimate AGB: the resulting maps have 10 m resolution, and uncertainties of usually less than 10%.
What sort of oversight/quality assurance practices are necessary to ensure the LTRMS functions properly and that the identification and quantification of reversals in the postcrediting period is accurate?
To ensure that the LTRMS functions properly, all data must be made publicly available and informed by the most up-to-date scientific information. The LTRMS could have oversight by Verra or an independent governance board if it is deemed necessary.
Learn how businesses are shaping carbon market strategies with carbon.

The end-to end carbon dataplatform for the 'net' in yournet zero goals
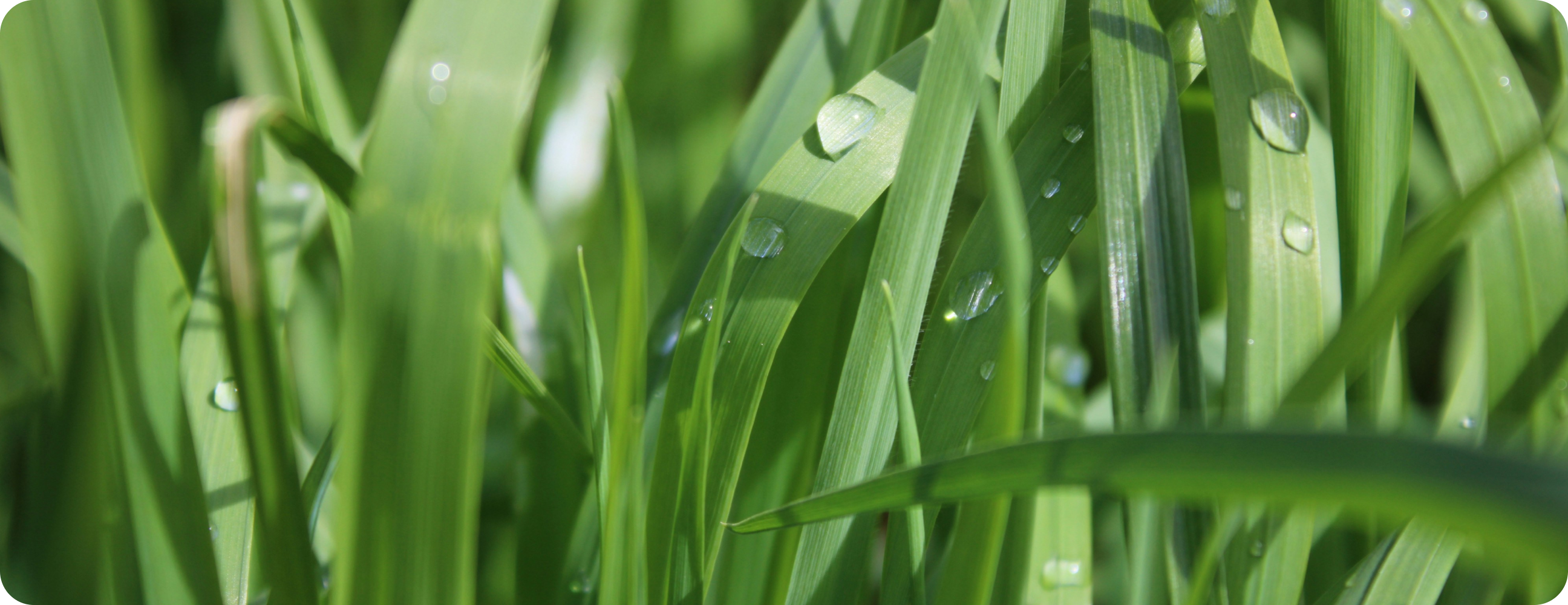